
Mike Howard
Mike has over 15 years of experience tracking the DRAM and memory markets. Prior to TechInsights, he built the DRAM research service at Yole. Prior to Yole, Mike spent time at IHS covering DRAM and Micron Technology where he had roles in engineering, marketing, and corporate development. Mike holds an MBA from The Ohio State University and a BS in Chemical Engineering and BA in Finance from the University of Washington.

Murali Emani
Murali Emani is a Computer Scientist in the Data Science group with the Argonne Leadership Computing Facility (ALCF) at Argonne National Laboratory. At ALCF, he co-leads the AI Testbed where they explore the performance, efficiency of novel AI accelerators for scientific machine learning applications. He also co-chairs the MLPerf HPC group at MLCommons, to benchmark large scale ML on HPC systems. His research interests are in Scalable Machine Learning, AI accelerators, AI for Science, and Emerging HPC architectures. His current work includes
- Developing performance models to identifying and addressing bottlenecks while scaling machine learning and deep learning frameworks on emerging supercomputers for scientific applications.
- Co-design of emerging hardware architectures to scale up machine learning workloads.
- Efforts on benchmarking ML/DL frameworks and methods on HPC systems.
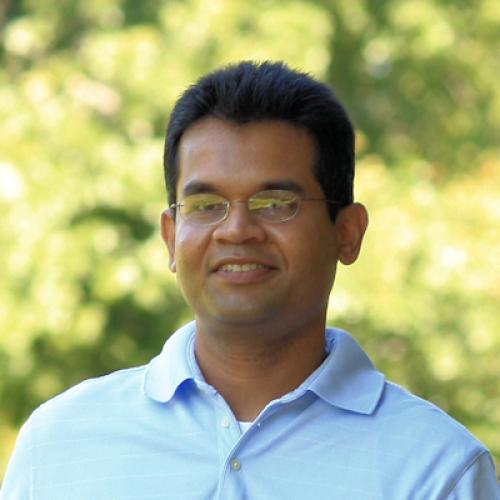
Nuwan Jayasena
Nuwan Jayasena is a Fellow at AMD Research, and leads a team exploring hardware support, software enablement, and application adaptation for processing in memory. His broader interests include memory system architecture, accelerator-based computing, and machine learning. Nuwan holds an M.S. and a Ph.D. in Electrical Engineering from Stanford University and a B.S. from the University of Southern California. He is an inventor of over 70 US patents, an author of over 30 peer-reviewed publications, and a Senior Member of the IEEE. Prior to AMD, Nuwan was a processor architect at Nvidia Corp. and at Stream Processors, Inc.

Simone Bertolazzi
Simone Bertolazzi, PhD is a Senior Technology & Market analyst, Memory, at Yole Intelligence, part of Yole Group, working with the Semiconductor, Memory & Computing division. As member of the Yole’s memory team, he contributes on a day-to-day basis to the analysis of memory markets and technologies, their related materials, device architectures and fabrication processes. Simone obtained a PhD in physics in 2015 from École Polytechnique Fédérale de Lausanne (Switzerland) and a double M. A. Sc. degree from Polytechnique de Montréal (Canada) and Politecnico di Milano (Italy), graduating cum laude.