About the Author
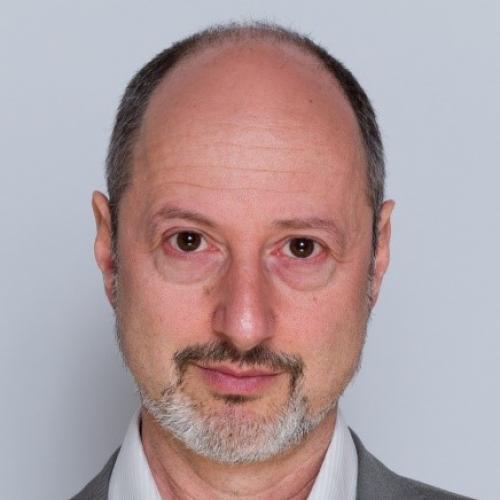
Michael Azoff
With over 17 years analyst experience, most recently at Ovum/ Informa, Michael Azoff joined Kisaco Research, the company behind the AI Hardware and Edge AI Summit series, in 2020 as Chief Analyst.
Eitan Michael Azoff, PhD, MSc, BEng.
HQ’d in Kisaco Research’s London office, Michael's current focus is launching Kisaco Research vendor product comparison reports with the new Kisaco Leadership Chart (KLC) analyst chart. The first KLC is also the first analyst chart in the AI chip industry, with 16 vendors having participated in the research.
In his career Michael worked at Rutherford Appleton Laboratory building simulators for electron and hole transport in semiconductors for UK national and European community research projects and published papers in learned journals. He then turned to building neural networks and created a startup selling his Prognostica Microsoft Excel add-in for time series forecasting, and wrote a book on the topic for publisher John Wiley & Sons in 1994.
Since 2003 Michael has worked as an IT industry analyst covering software engineering topics, from agile and DevOps, to application lifecycle management and cloud native computing. He started covering machine learning when deep learning emerged as the most recent wave of interest in AI and left his position as Distinguished Analyst at Ovum/Informa to join Kisaco Research and help build an analyst capability within the company.
My analyst coverage areas at KR Analysis
My first research project at KR was to create the first analyst comparison chart for AI chips. We invited AI chip producers to participate and were fortunate to have 16 vendors participate from across the globe: USA, UK, France, and China, and a mix of established players (Nvidia, Imagination, Intel, and Xilinx, to startups.
Our analysis showed that the market naturally fell into three areas of hot activity:
▪ Data centers and high-performance computing environments (HPC): here large boxes are installed and the aim is to achieve maximum performance for training and inferencing AI systems. The buyers are cloud hyperscalars, national research labs and agencies, and some large enterprises with big investments in AI.
▪ Small edge: the opposite end of the spectrum, building the smallest useful chip possible to sell as cheap as possible and embed in edge devices. AI is inferencing here.
▪ Automotive: an active industry in AI but highly regulated creating hurdles and technology adoption cadences that can be challenging for suppliers. AI is mainly inferencing here (for systems installed in vehicles).
We produced four Kisaco Leadership Charts out of this research.
We are also researching the machine learning (ML) software tools space, and our first report here is ML Lifecycle Solutions. The biggest challenge for enterprises is taking the research AI systems developed by their data scientist and deploying these into production at scale. Using a host of open source tools to achieve this is possible but time consuming to build and maintain, as well as prone to breakdown. This is why the ML lifecycle solution space exists.
Finally, in our first batch of KR Analysis reports we produced the KLC on engineering application lifecycle management (ALM) solutions. While ALM has been in existence as a distinct practice since KR Analysis and Michael Azoff introduction © Kisaco Research. All rights reserved. Unauthorized reproduction prohibited. 4 around 2003, it continues to evolve. We found the engineering and highly regulated industries relying on engineering and compliance oriented ALM to help manage risk and complexity.
-
Motivation
Today Artificial intelligence (AI) is out of the research laboratory and in the realm of practical engineering applications. AI engineering today is largely about running machine learning (ML) models on digital computers, and these models are typically simulations of brain-inspired models such as neural networks, with deep learning (DL) being the most successful example today. With the plateauing out of CPU performance improvements and the end of Moore’s law, even with multi-core CPU machines, the community has turned to hardware accelerators to run their AI models.
While the cloud has become the marker for our current age of computing, the edge is set to take over the limelight and the most straight forward reason is that it is where most of the data is generated and we are moving to technology that can process it at source rather than create lag and throughput bottlenecks in shifting it first to the cloud.
The AI hardware accelerators needed for edge computing and for the automotive market are in stark contrast to those needed in the data center (DC) and for high performance computing (HPC). Whereas in the DC AI models are typically trained and inferenced, on the edge AI models are typically just inferenced (training can be done at the edge but the chips we review are designed for the most common use case of edge inferencing). Size and power constraints are also significant factors in the edge which have less effect in DC/HPC choices.
The edge represents a spectrum of use cases and so we focus on small sized chips for small edge scenarios such as embedded AI in consumer products, security systems, sensors, and a host of smart devices. The automotive market, which has distinct requirements from other edge computing, also covers a spectrum of use cases in the vehicle: smart controllers, ADAS, and autonomous vehicles (AV). We focus on AI chips suitable for the AV market. Thus, this report contains a KLC for each of these market segments: small edge and automotive-AV.
In this report Kisaco Research provides two Kisaco Leadership Charts (KLCs) 2020-21: one for Small Edge AI inferencing and one for Automotive-AV AI inferencing, with full profiles and assessments of all participating vendors.
-
What you will learn
- The attributes of the edge computing and automotive application markets and how they differ from the rest of the market.
- Who are the key players in the edge & automotive market, with deep profiles of ten of our participating vendors competing in AI inferencing, including strengths and weaknesses.
- Two analyst charts, the Kisaco Leadership Chart (KLC), on the participating vendors: one for small edge AI inferencing and one for automotive AI inferencing. These are the first such charts ever produced in the AI chip market.
- What are the attributes of AI chips in the edge space versus other use cases in the market. Variables such as energy consumption, cost, precision and more.
- Why the automotive market is a distinct challenge for AI chip manufacturers and the impact of working in a highly regulated market.
-
Contents
Kisaco Research View. 2
Motivation. 2
Definitions are important 2
AI 2
The small edge. 3
Key findings. 3
Companion reports. 4
Solution analysis: AI inferencing on the edge. 4
Technology and market trends. 4
Market segments. 4
Small edge. 5
Autonomous driving. 7
AI accelerator power, size, and cost constraints. 8
Example small edge application: keyword spotting. 10
Solution analysis: vendor comparisons. 11
Kisaco Leadership Chart on AI hardware accelerators 2020-21: edge and automotive. 11
Introduction. 11
The KLC charts for AI hardware accelerators: AI inference for small edge. 12
The KLC charts for AI hardware accelerators: AI inference for automotive-AV. 15
Data centers and HPC (a companion report) 17
Vendor analysis. 17
Eta Compute, Kisaco evaluation: Emerging Player 17
Kisaco Assessment 20
GrAI Matter Labs, Kisaco evaluation: Innovator 20
Kisaco Assessment 23
Hailo, Kisaco evaluation: Emerging Player 24
Profile. 24
Kisaco Strengths and Weaknesses Assessment 26
Horizon Robotics, Kisaco evaluation: Leader 27
Profile. 27
Kisaco Assessment 29
Imagination Technologies, Kisaco evaluation: Leader 30
Kisaco Assessment 32
Kalray, Kisaco recommendation: Innovator 32
Kisaco Assessment 35
Kneron, Kisaco evaluation: Contender 35
Kisaco Assessment 38
Mythic, Kisaco evaluation: Innovator 38
Kisaco Assessment 41
Nvidia, Kisaco evaluation: Leader 42
Kisaco Assessment 46
Syntiant, Kisaco evaluation: Contender 46
Kisaco Assessment 49
Tsingmicro, Kisaco evaluation: Contender 49
Kisaco Assessment 51
Appendix. 52
Vendor solution selection. 52
Inclusion criteria. 52
Exclusion criteria. 52
Methodology. 52
Definition of the KLC. 52
Kisaco Research ratings. 53
Further reading. 53
Acknowledgements. 53
Author 53
Copyright notice and disclaimer 53
-
Figures
Figure 1: Market segments AI training and inferencing characteristics
Figure 2: Convergence of technologies and impact on edge applications
Figure 3: Convergence of technologies and impact on edge applications
Figure 4: ISO 26262 part 6: error handling methods by ASIL rating: ++ = highly recommended, + = recommended, 0 = no recommendation
Figure 5: SAE driving automation levels defined
Figure 6: Example constraints at the edge with some typical values
Figure 7: The reduction in fabrication process size by year
Figure 8: Accuracy vs. memory and operations of different ML models
Figure 9: Market segment applications for AI hardware accelerators
Figure 10: Kisaco Leadership Chart on AI Hardware Accelerators 2020-21: small edge – AI inference
Figure 11: Kisaco Leadership Chart on AI Hardware Accelerators 2020-21: small edge – AI inference: ranking of vendors
Figure 12: Kisaco Leadership Chart on AI Hardware Accelerators 2020-21: automotive-AV – AI inference
Figure 13: Kisaco Leadership Chart on AI Hardware Accelerators 2020-21: automotive-AV – AI inference: ranking of vendors
Figure 14: Eta Compute’s patented technology CVFS improves over traditional DVFS
Figure 15: Eta Compute ECM3532, neural sensor processor with CVFS
Figure 16: GML GrAI One architecture
Figure 17: Hailo-8: structure defined dataflow architecture
Figure 18: Horizon Robotics, BPU core based on heterogeneous MIMD architecture
Figure 19: Imagination Technologies PowerVR AI software stack
Figure 20: Kalray MPPA architecture
Figure 21: Kneron NPU architecture
Figure 22: Mythic exploits embedded Flash transistors as variable resistors to hold weights
Figure 23: Mythic DNN chip with deep learning neural network tiled architecture
Figure 24: Nvidia GA100 GPU with 128 SMs – with strips removed to provide legibility
Figure 25: Nvidia: Internals of a GA100 SM
Figure 26: A100 GPU performance in BERT deep learning training and inference modes comparing Tesla V100 and Tesla T4
Figure 27: Syntiant NDP100 architecture
Figure 28: CGRA in the context of other processor architecture types
Figure 29: Tsingmicro: the compiler configures the PE functions (orange squares) and data flow in real-time
-
FAQs
1. What is the KLC?
The Kisaco Leadership Chart (KLC) is KR Analysis’s take on the classis industry analyst chart in which vendor products are assessed and their scores plotted on a chart comprising four quadrants: Leader, Contender, Innovator, and Emerging Player. The x-axis represents strength of technical features, the y-axis the strength of market execution and strategy, and the size of plotted circle represents market revenue normalized to the strongest participating player in the research.
In researching the KLC we receive privileged information from a vendor. As explained in question 3, participating vendors are actively engaged in our research. Confidential privileged vendor information is not disclosed in our report but helps us assess vendors in our analysis.
2. What is the vendor selection process for a KLC project?
KR Analysis creates a shortlist of vendors to invite to the research project. The aim is to include the leading players as well as innovative smaller players, across startup and established vendors. KLC research can at best be representative of the market and is not designed to be exhaustive – in some markets the sheer number of players would make an exhaustive KLC unmanageable, in smaller markets we are still dependent on vendors agreeing to participate.
We do create KR Analysis Technology and Market Landscape reports in which we typically list the players in the markets with thumbnail profiles providing information such as company leadership, location, funding status, and main product(s) details. While we cannot guarantee exhaustiveness, the landscape report does aim to list the most important vendors and does not require vendor participation.
3. In a KLC, what does participating entail for a vendor?
First of all, we do not charge vendors to participate in a KLC. Participating vendors need to be actively engaged in a KLC research project, this involves completing a comprehensive questionnaire, which we score and use as the basis for positioning the vendor in the report’s KLC. We also hold a deep dive briefing and engage in plenty of Q&A. Finally, we research publicly available material on the vendor and its product(s) to complete our final view of the vendor.
4. Why are some notable vendors missing from the report?
As explained in question 2, we do invite the leaders in a market segment we are researching, however not all such players agree to participate. As explained in question 3, participating involves active engagement and example reasons vendors offer for declining our invitation are, often ending with “...but please consider us next cycle of the report.”:
- We are in the midst of an event in which our relevant staff do not have the time to engage in your process.
- We are going through a major change in strategy or product re-architecture and the timing is not right for us to participate.
- We are about to have our IPO and this is not the right time to participate.
- We are about to launch our flagship product and the report timing is not right for us.
-
About the Author
Michael Azoff
Chief AnalystKisaco ResearchWith over 17 years analyst experience, most recently at Ovum/ Informa, Michael Azoff joined Kisaco Research, the company behind the AI Hardware and Edge AI Summit series, in 2020 as Chief Analyst.
Eitan Michael Azoff, PhD, MSc, BEng.
HQ’d in Kisaco Research’s London office, Michael's current focus is launching Kisaco Research vendor product comparison reports with the new Kisaco Leadership Chart (KLC) analyst chart. The first KLC is also the first analyst chart in the AI chip industry, with 16 vendors having participated in the research.
In his career Michael worked at Rutherford Appleton Laboratory building simulators for electron and hole transport in semiconductors for UK national and European community research projects and published papers in learned journals. He then turned to building neural networks and created a startup selling his Prognostica Microsoft Excel add-in for time series forecasting, and wrote a book on the topic for publisher John Wiley & Sons in 1994.
Since 2003 Michael has worked as an IT industry analyst covering software engineering topics, from agile and DevOps, to application lifecycle management and cloud native computing. He started covering machine learning when deep learning emerged as the most recent wave of interest in AI and left his position as Distinguished Analyst at Ovum/Informa to join Kisaco Research and help build an analyst capability within the company.
My analyst coverage areas at KR Analysis
My first research project at KR was to create the first analyst comparison chart for AI chips. We invited AI chip producers to participate and were fortunate to have 16 vendors participate from across the globe: USA, UK, France, and China, and a mix of established players (Nvidia, Imagination, Intel, and Xilinx, to startups.
Our analysis showed that the market naturally fell into three areas of hot activity:
▪ Data centers and high-performance computing environments (HPC): here large boxes are installed and the aim is to achieve maximum performance for training and inferencing AI systems. The buyers are cloud hyperscalars, national research labs and agencies, and some large enterprises with big investments in AI.
▪ Small edge: the opposite end of the spectrum, building the smallest useful chip possible to sell as cheap as possible and embed in edge devices. AI is inferencing here.
▪ Automotive: an active industry in AI but highly regulated creating hurdles and technology adoption cadences that can be challenging for suppliers. AI is mainly inferencing here (for systems installed in vehicles).
We produced four Kisaco Leadership Charts out of this research.
We are also researching the machine learning (ML) software tools space, and our first report here is ML Lifecycle Solutions. The biggest challenge for enterprises is taking the research AI systems developed by their data scientist and deploying these into production at scale. Using a host of open source tools to achieve this is possible but time consuming to build and maintain, as well as prone to breakdown. This is why the ML lifecycle solution space exists.
Finally, in our first batch of KR Analysis reports we produced the KLC on engineering application lifecycle management (ALM) solutions. While ALM has been in existence as a distinct practice since KR Analysis and Michael Azoff introduction © Kisaco Research. All rights reserved. Unauthorized reproduction prohibited. 4 around 2003, it continues to evolve. We found the engineering and highly regulated industries relying on engineering and compliance oriented ALM to help manage risk and complexity.
Request a sample
Please complete the form below to receive a sample of this report.
Purchase the report
Single User | $4,999 | Buy Online
Company-wide Use | $5,999 | Buy Online
VAT will be added for companies based in the UK. For further information or support in purchasing a report, please contact email: [email protected]